Document Type
Article
Publication Title
Business Process Anomaly Detection and Root Cause Analysis Using BLSTM-VAE With Attention
Abstract
Detecting anomalous executions in business process data is crucial for safeguarding the efficiency and success of an organization. Unsupervised approaches are commonly used for business process anomaly detection because of the scarcity of labeled anomaly data. However, these approaches often encounter a notable decline in performance because they lack prior knowledge about the anomalies. Additionally, most of them do not perform root cause analysis on the detected anomalies. This study proposes a variational autoencoder-based approach to overcome the performance limitations of existing unsupervised methods and determine the root causes of the detected anomalies. The learning of the variational autoencoder from unlabeled business process data is enhanced in the proposed approach by leveraging different architectural components, namely, the entity embedding technique, the bidirectional long short-term memory network, and the self-attention mechanism. Combining these architectural components in the variational autoencoder architecture leads to learning high-level representations from the business process data and thus improving the reconstruction capability of the variational autoencoder. Furthermore, this study suggests feeding the reconstruction error provided by the variational autoencoder into the logistic regression classifier to improve the accuracy of anomaly detection. The performance of the proposed model was evaluated on real-life and synthetic datasets. The experimental findings indicate that the proposed model outperforms six existing anomaly detection models in terms of precision, recall, and F1-score metrics.
DOI
10.1109/ACCESS.2024.3406633
Publication Date
5-30-2024
Recommended Citation
Ismail, Yasser; El-Aziz, Eman; Fathalla, Radwa; and Shaheen, Mohamed, "Business Process Anomaly Detection and Root Cause Analysis Using BLSTM-VAE With Attention" (2024). Faculty Publications. 23.
https://digitalcommons.subr.edu/osp_facpubs/23
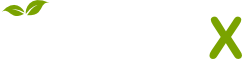
- Citations
- Citation Indexes: 2
- Usage
- Downloads: 43
- Abstract Views: 20
- Captures
- Readers: 5
- Mentions
- News Mentions: 1